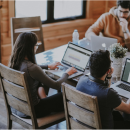
How data analytics and machine learning can be used to optimise property management, leasing, and commercial property investment decisions.
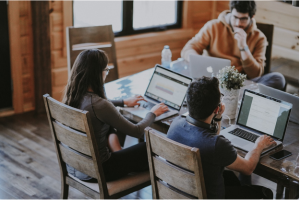
Photo by Annie Spratt on Unsplash
In the commercial property sector, the integration of data analytics and machine learning has become increasingly prevalent. This trend aims to optimise various aspects of property management, leasing, and investment decision-making. By harnessing data-driven insights, professionals in the commercial property industry can make more strategic and well-informed choices.
A key area where data analytics and machine learning can contribute is in the enhancement of property management. Through the collection of real-time data from sensors, IoT devices, and other sources that monitor building performance, opportunities for improving efficiency and tenant satisfaction can be identified:
- Predictive Maintenance: By analysing collected data, it becomes possible to predict when equipment or facilities will require maintenance. This proactive approach allows property managers to schedule maintenance in advance, reducing the likelihood of costly emergency repairs and downtime.
- Condition-based Maintenance: Data analysis helps property managers identify trends and patterns in equipment performance. This information can inform condition-based maintenance schedules, which involve addressing maintenance needs based on indicators suggesting a decrease in performance. For instance, if a piece of warehouse machinery begins to overheat, appropriate maintenance actions can be taken.
- Energy Management: Property managers can utilise data analysis to identify areas of high energy consumption. By taking steps such as replacing outdated equipment, improving insulation, or adjusting temperature settings, energy usage can be reduced, leading to lower costs.
- Asset Management: Data analysis empowers property managers to track all assets, including equipment, facilities, and systems efficiently.
Lease optimization is another valuable application of data analytics and machine learning in the commercial property sector. By analysing market trends and tenant data, commercial property owners can determine optimal lease terms and current rental rates for their properties. Additionally, advanced algorithms can identify the ideal tenant profile for a landlord’s specific property type, facilitating the selection process.
Once tenants are in place, machine learning can monitor their behaviour and preferences. Multiple data sources, such as surveys, social media, building sensors, and demographic data, can be utilised to gather valuable insights. This comprehensive data helps landlords identify the amenities and services that will effectively retain their chosen tenants.
Furthermore, data analytics and machine learning play a vital role in property investment decisions. Historical transaction data and market trends enable the identification of investment opportunities and the evaluation of potential risks and returns associated with specific property types. Real-time analysis of financial and market data by machine learning algorithms empowers investors to make well-informed and timely decisions.
However, it is essential to acknowledge the limitations and challenges associated with using AI in this context. Potential challenges include data bias arising from non-representative samples or unaccounted variables, as well as unintentional biases introduced by data analysts. To effectively interpret and act on the insights provided by these technologies, commercial property professionals and businesses must possess the necessary digital skills and knowledge.
Lastly, high-quality data input is crucial. The data should be accurate, complete, reliable, relevant, and timely. By leveraging real-time data and insights, stakeholders in the commercial property industry can make more informed and strategic decisions. This, in turn, leads to improved efficiency, tenant satisfaction, and investment returns. Nonetheless, it is important to consider the limitations and challenges associated with these technologies and to invest in the skills and knowledge required to effectively leverage them in the industry.